What NIST Data Shows About Facial Recognition and Demographics
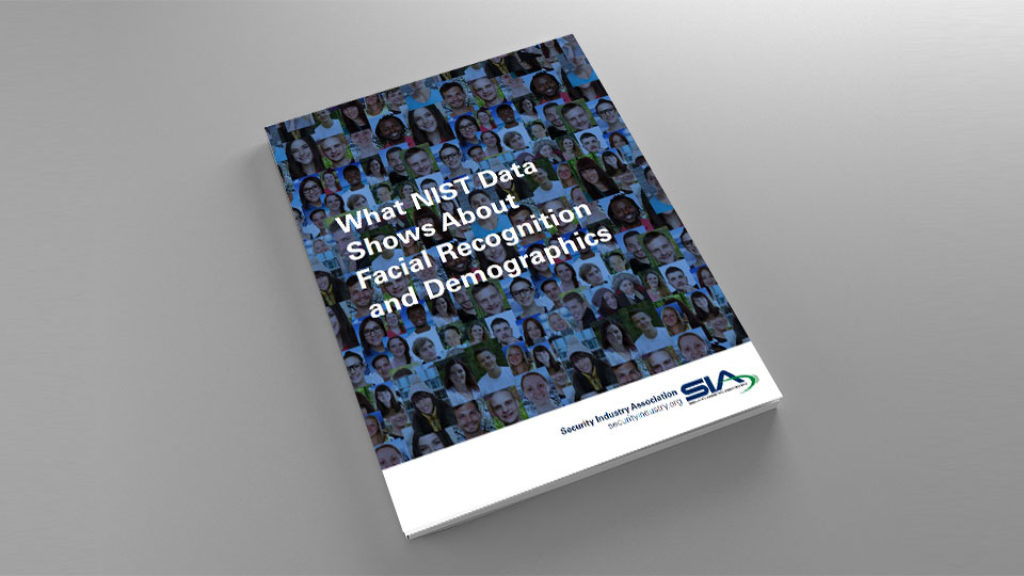
In December 2019, the National Institute of Standards and Technology (NIST) published the most comprehensive report to date on the performance of facial recognition algorithms – the core component of facial recognition technology – across race, gender and other demographic groups. The most significant takeaway from the NIST report is that it confirms current facial recognition technology performs far more effectively across racial and other demographic groups than had been widely reported; however, we’ve seen some misleading conclusions drawn from the highly technical 1,500-page report. A closer look at the findings in their proper context is essential to understanding the implications.
Key Takeaways
- Facial recognition technology performs far more effectively across racial and other demographic groups than widely reported.
- The most accurate technologies displayed “undetectable” differences between demographic groups, calling into question claims of inherent bias.
- Key U.S. government programs are using the most accurate technologies.
- Accuracy rates should always be considered in application-specific contexts.
Role of NIST in Facial Recognition Evaluation
For the past 20 years, NIST’s Face Recognition Vendor Test (FRVT) program has been the world’s most respected evaluator of facial recognition algorithms – examining technologies voluntarily provided by developers for independent testing. NIST’s December report is the most comprehensive scientific evaluation to date of current facial recognition technology performance across demographic variables, involving 189 algorithms from 99 developers using 18 million images of 8 million people within four different data sets. The results are a snapshot in time, providing a critical benchmark against which developers work to improve the technology, as industry progress is tracked through the ongoing FRVT program.
Purpose of the Report and What It Found
NIST’s report addresses “assertions that demographic dependencies could lead to accuracy variations and potential bias” as well as flaws in prior research and media reporting. “Much of the discussion of face recognition bias in recent years cites two studies showing poor accuracy of face gender classification algorithms on black women. Those studies did not evaluate face recognition algorithms, yet the results have been widely cited to indict their accuracy,” according to the report. The most-cited figure from those papers is that two such algorithms assigned the wrong gender to photos from that demographic group nearly 35 percent of the time. This was reported widely in media reports as a groundbreaking discovery on facial recognition accuracy even though it did not even assess this technology.
In contrast, NIST found that, “To the extent there are demographic differentials, they are much smaller,” pointing out error rates in verification-type algorithms are “absolutely low,” generally below 1 percent and many below 0.5 percent. Even more significantly, NIST found that in the most accurate across demographic groups were “undetectable.” It would not be possible to mitigate these effects if bias is inherent in facial recognition technology, as some have alleged.
Notably for policymakers, the most well-known U.S. government applications already use some of the highest performing technologies. The report specifically identifies six suppliers of identification-type algorithms with undetectable differences in “false positive” rates. Included among these are current technology suppliers to the Federal Bureau of Investigation Criminal Justice Information Services Division and U.S. Customs and Border Protection’s Traveler Verification Service.
For the rest of the algorithms, the report found that higher overall accuracy means smaller differences in performance across demographic groups. NIST did find relatively higher false positive effects for some groups in the majority of algorithms tested – depending on the specific metric, type of algorithm, chosen similarity score threshold and data set involved. However, as one recent analysis of the report noted “Algorithms can have different error rates for different demographics but still be highly accurate.”
NIST charts comparisons across demographic groupings on a logarithmic scale because this granularity allows us to better perceive relative differences between error rates produced by algorithms that may be highly accurate in absolute terms. According to NIST, “readers don’t perceive differences in numbers near 100% well,” due to the “high nineties effect where numbers close to 100 are perceived indifferently.”
As a result, some figures in the report appear large if considered only in relative terms. Using photos from over 24 countries in seven distinct global regions, verification-type algorithms produced false match rates for photos of individuals originally from East Africa as much as “100 times greater than baseline.” Although performance variations across demographic groups are important to continually assess and critically examine, outside of Somalia nearly all country-to-country comparisons across algorithms yielded false match rates of less than 1 percent despite the magnitude of differences identified.
Similarly, only four out of 116 algorithms tested using the U.S. Mugshot Identification Database had false match rates of more than 1 percent for any demographic: male, female, black, white, Asian or American Indian. One example cited by NIST produced a 0.025% false match rate for black males and a 0.1% false match rate for black women. Compared to the rate for white males, this is 10 times higher for black women and 2.5 times higher for black males; however, these error rates are at or below one tenth of one percent.
Certainly, significant gaps were found between the very highest- and lowest-performing algorithms. NIST tests any algorithm submitted and many of these are in the early stages of development. Lower-performing technologies are less likely to be deployed in commercial products.
Accuracy in Context
There will always be error rates for any biometric, or any technology for that matter. For example, this is why NIST compared false match rates for different demographic groups to each other, not zero. How is accuracy defined when it comes to demographic effects? According to NIST, it means these rates “do not vary (much) over any demographics.”
Overall, modern facial recognition technology is highly accurate. It is in fact image quality variations like pose, illumination and expression have been the primary driver of errors in facial recognition performance, not demographic effects, and growing immunity to such problems is, according to NIST, the “fundamental reason why accuracy has improved since 2013.”
NIST has documented massive improvements in recent years, noting in 2018 the software tested was at least 20 times more accurate than it was in 2014, and in 2019 finding “close to perfect” performance by high-performing algorithms with miss rates averaging 0.1 percent. On this measurement, the accuracy of facial recognition is reaching that of automated fingerprint comparison, which is generally viewed as the gold standard for identification.
Lab Tests vs. Real World
We simply aren’t seeing instances in the U.S. where demographic performance differences in widely used algorithms are affecting facial recognition systems in high-risk settings. There are several reasons that may explain why.
Algorithms comprise just one of several components of facial recognition systems. A human analyst will play a critical role in use of facial recognition as a tool in law enforcement investigations or as part of any process with potential high-consequence outcomes for individuals. There are no automated decisions made solely by technology in these cases. Personnel adjudicates in situations where the technology may not work as well as intended. NIST has documented that the most accurate identification results occur when facial recognition is combined with trained human review, versus either element alone. This may explain U.S. law enforcement’s decade-plus operating history without any example of it contributing to a mistaken arrest or imprisonment.
False positives are naturally limited by the size of the data set used. A larger set of photos likely has a larger number of similar people in it; however, for many applications, the data sets are relatively small – the 250 passengers on a flight or two dozen people authorized to enter a building, for example, which will naturally limit false positives.
NIST calls for considering different accuracy measurements within the context of the “performance metric of interest” for specific applications, noting the study is the first to “properly report and distinguish between false positive and false negative effects.” The real-world implications of each depend entirely upon the specific use and mitigating factors. An error could be mostly inconsequential in cases where a “subject experiencing a false rejection could make a second attempt at recognition” in order to unlock a door or device or clear passport control, for example.
One of the report’s key findings was that false positive rates vary much more across demographic groups than false negative effects; however, false negative effects are more critical to many uses identified. For example, facial recognition is used to detect fraud attempts when the same person applies for driver’s license applications under different identities, ensuring this person is not the same as any other in a database. This is also how it works in many security applications, where the purpose of photo comparison is to ensure persons entering a building do not match those on a persons of interest list. In both cases, the false negative rate is the key performance measurement because the antifraud or security objective requires a very low likelihood of missing a possible match to flag for human review.
For law enforcement investigations, ensuring that possible matches are not missed is even more critical. According to the NIST report, “false positive differentials from the algorithm are immaterial” for law enforcement investigations since all searches produce a fixed number of candidates for human review regardless of any threshold for similarity score. On the other hand, at a port of entry, there may be a relatively high risk of persons attempting to enter under another identity, so false positive effects may be more critical. In a low-risk application like entry to an amusement park, both accuracy measurements may be less critical due to the low probability of someone trying to impersonate someone with a ticket and the operational need to speed entry by limiting rejections.
Limitations of the Report
Despite taking the most comprehensive look so far at demographic effects in facial recognition performance, the NIST report does have limitations and raises some unanswered questions. Most significantly, it is not clear whether ethnicity was fully isolated from other demographics or capture conditions in many instances. For example, false match rates for Somalia are very significant outliers that are not fully explained. These error rates are far higher for Somalians than neighboring countries in nearly every algorithm tested. One of the most accurate verification algorithms overall had a false match rate of about 1 percent for Somalia, while for neighboring Ethiopia – which has a closely related ethnic majority – it was just 0.07 percent, more than 14 times lower. This dramatic difference would suggest that the impact of ethnicity was not isolated and that other differences, in capture conditions, data labeling errors, etc. between country data exist.
Implications for the Security Industry
Applied to security solutions developed by our industry, biometric technologies like facial recognition increase the effectiveness of safety and security measures that protect people from harm. Any significant bias in technology performance makes it harder to achieve this goal.
We understand that there are legitimate concerns that use of facial recognition technology might negatively impact women and minorities. Industry is striving to provide technology that is as effective and accurate as possible across all types of uses, deployment settings and demographic characteristics in order to fully address these concerns.
Both developers and end users have a responsibility to minimize any negative effects that could result when the technology does not perform as intended though proper design, configuration, policies and procedures. We strongly believe that facial recognition makes our country safer and brings value to our everyday lives when used effectively and responsibly. No technology product should ever be used for purposes that are unlawful, unethical or discriminatory.